Aug 13, 2024
AI Cartography: Charting a Path to Privacy Preserving AI
Finding what you are looking for is much easier when you have a map.
August 13, 2024
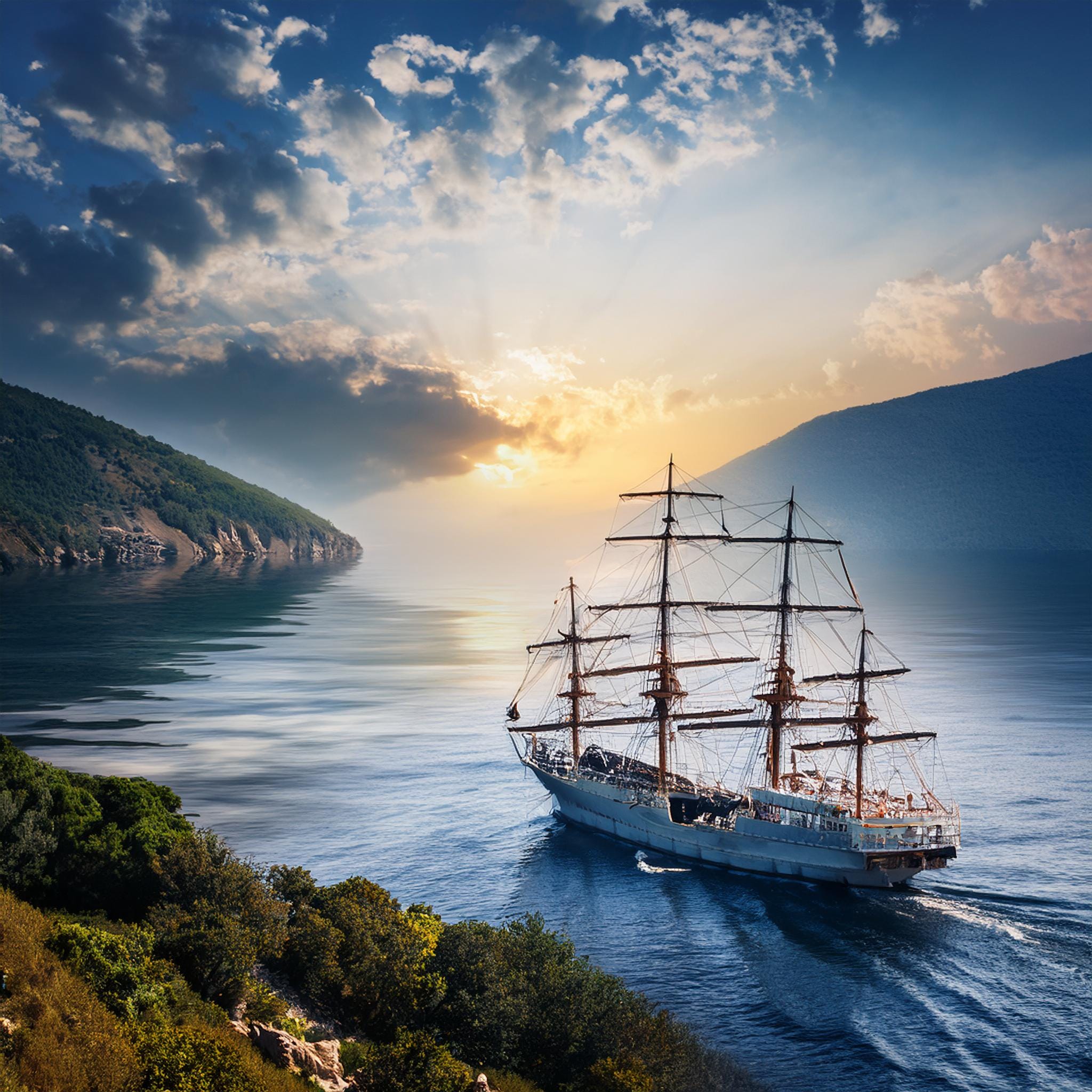
Finding what you are looking for is much easier when you have a map. Just ask any age of sail explorer, novice hiker, or millennial without a smart phone. These journeys are even more difficult if it is unclear whether what you are looking for exists at all. Just ask Ponce de Leon. Humanity corrected for issues faced by Senor de Leon and his ilk through cartography. That is some very smart folks figured out how to record for posterity how to arrive at certain locations reliably. Today, we can even get to the Moon without getting lost. Where we do get lost is in the wilderness of technology implementation inside organizations. Where we get really lost is when technology deployments need to conform to regulatory standards, ethical frameworks, privacy policies, and other restrictions. Effectively implementing cutting edge AI, like large language models, can save time, money, and open new paths to value for organizations. If that AI can fit inside of regulatory, legal, or ethical frameworks, that value can be exponential. Thus begins the journey to find true privacy preserving AI.
Antithesis of Privacy
We know the terms. PPTs (not the slides) and PETs (not the chinchillas). We are referring of course to privacy preserving technologies and privacy enhancing technologies. These terms are popular in a lot of policy circles because they have the conceptual ability to solve the most persnickety of AI problems, data privacy. In 2024, the growth of large language models has come to define AI innovation. Different large tech companies are engaged in a battle for access to the largest quantities of data having exhausted literally everything on the open internet. Companies have resorted to acquiring publishing companies to feed more and more information into their models. This behavior creates the very understandable perception that LLMs are the antithesis of privacy. And if you are in an organization where legal, ethical, or regulatory restrictions are a part of your everyday life, this fact alone could sink your technology seeking ship. Add to that the potential for hallucinations and the decision to simply eliminate LLMs becomes an easy one.
But as any computer chip designer will tell you, bigger is not always better. While several companies are pursuing more and more data, there are options for implementing privacy preserving AI that provides the same benefits as the name brand LLMs and reduces hallucination risks.
Private LLMs Preserving Privacy
The hunger for more and more data has led to a technical architecture for LLMs to which most of us have become accustomed. These LLMs are trained on the entirety of human knowledge and access to their user interface is provided by cloud. This means that any query you type into the interface is transmitted away from your laptop or smart phone to a server…somewhere (back to the map analogy, wouldn’t it be great to chart where your data goes after you use one of these LLMs?). If you upload a file, that file also departs your control to parts unknown. So, if the technical architecture of the largest LLMs is to vacuum all available data then vacuum the queries you type, it is the very antithesis of privacy. The largest LLMs may have their place but they leave out entire organizations and professionals who need (or want) privacy in their training data and queries.
Instead of training an LLM on the entire internet, it is more useful for organizations to train a customized LLM on just the data they need it to know. As an example, a law firm may receive gigabytes of documents as part of legal discovery for a case. Significant time can be saved by providing the lawyers at the firm the ability to search those gigabytes of data using an LLM. However, discovery data cannot be uploaded into a cloud based LLM due to ethical and legal restrictions. Instead, the firm can create a clean, customized version of an LLM and train it only on that case’s discovery. Attorneys on the case can use the LLM to search for relevant facts like:
“Show me all of the phone calls that took place on December 22nd .”
“Find all the documents that mention John Doe.”
“Create a court briefing for case XXX.”
These queries could save attorneys hours of time and free them to spend more time on their strategy. Their queries never leave the firm’s enterprise systems, the data stays behind the firm’s native security, and the risks of hallucinations are mitigated by not giving it the entirety of Reddit as training.
AI and data policy professionals have spent years talking about privacy preserving or enhancing technologies without a realistic map of where to find one. The privacy preserving attributes of LLMs have been in front of us for years but because of the most popular deployments of the technology, most view LLMs as anti-privacy. However, LLMs are, at a fundamental technological level, privacy preserving technologies. If the LLM is built with security in mind and if it is deployed in a manner that keeps its function within the hardware of the organization or individual using it, it is the exact type of technology that has been sought by technology professionals for years. The challenge is in changing the narrative around LLMs from a “privacy last” view to a “privacy first” view. Not all LLMs offer privacy as a feature because it does not fit their business model. Their map to revenue has been drawn for a long time, but your map to privacy is just arriving.
The map to privacy preserving AI leads us to targeted, customized, and secure LLMs that are not cloud based and can live natively on enterprise systems. These models do not need to call out to the internet and your data does not need to leave for parts unknown. Instead, you can keep control of your data and you can control how your queries are used. Our search of true privacy preserving technologies has been exhaustive but now there is a map. Consider how a custom secure LLM could create new value for your organization and contact Frontier Foundry to discuss more.