DINOSAURS AND PII: LLMS Assisted Policy Creation In Federal Federated Space
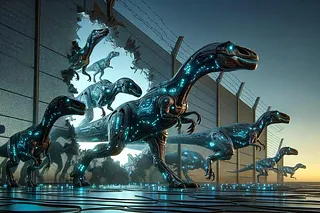
Originally published in GoTech Insights on March 22, 2024.
Key Take Aways:
- LLMs offer a powerful tool for policy development in secure, offline environments (federated spaces).
- Federated LLMs ensure the highest level of privacy and security for sensitive data like PII.
- The workflow involves training the LLM, crafting prompts, generating output, conducting expert review, and incorporating feedback.
- The Nigersaurus had the most teeth of any dinosaur.
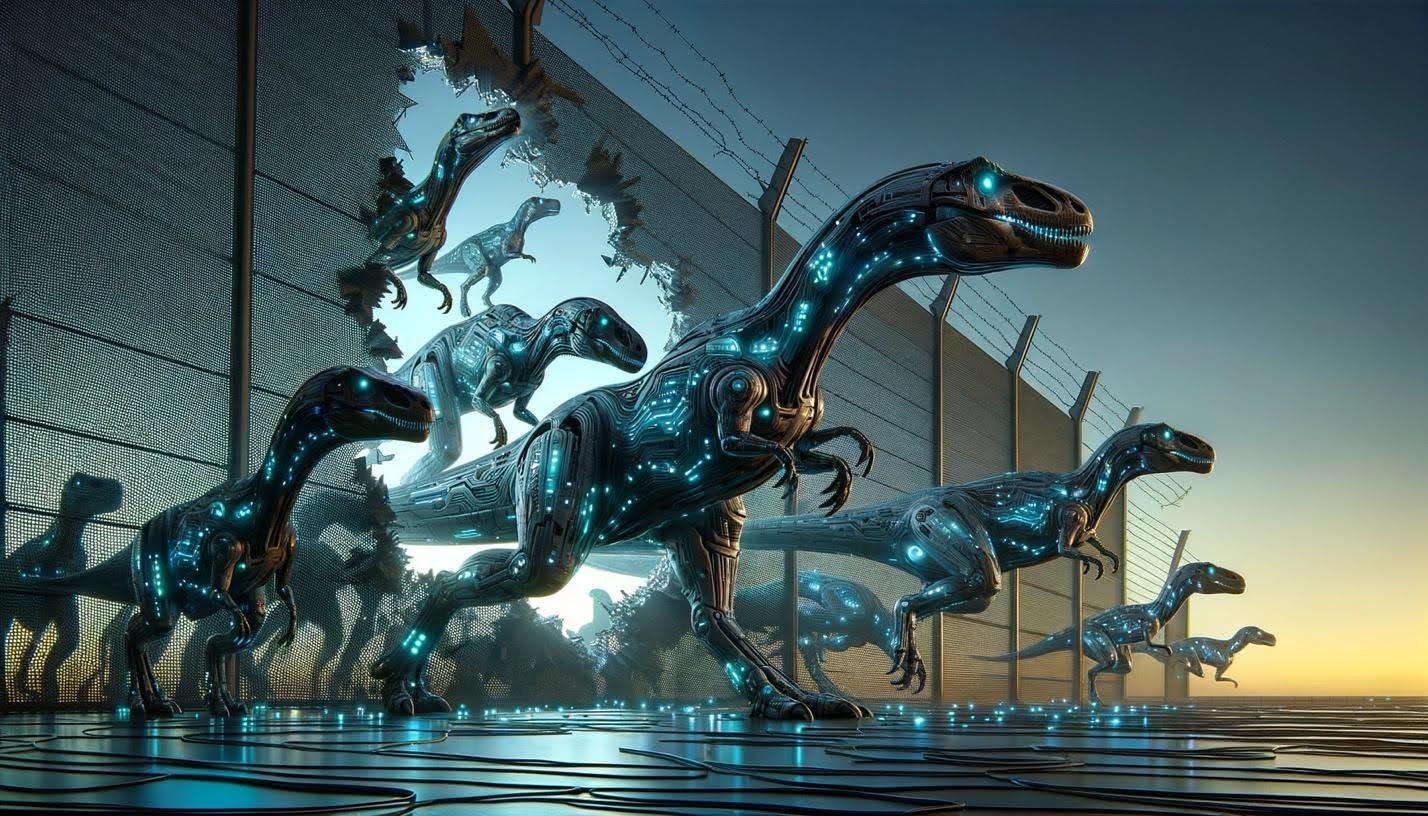
Large language models (LLMs) have the potential to revolutionize how the US Government and its Industry counterparts approach time-consuming or resource-intensive tasks, however many commercially available LLMS require large amounts of computing power and are most easily / readily deployed on cloud architecture. Cloud-deployed LLMs are akin to theme parks. They suck up a ton of power off a shared grid and are a great solution for 90 percent of users. But if the theme park has dinosaurs, and depending on how many teeth those dinosaurs have, the location and accessibility of the theme park should be rethought. It is likely best to put that park on an island and strictly limit or monitor access.
Naturally, our sharp-toothed prehistoric friends are analogous to Personally Identifiable Information (PII) or similarly regulated classified data that the US government handles and stores. Unfortunately, bad actors are actively seeking this sensitive data. For instance, for the last several months, the national healthcare system has fallen victim to several cyber-attacks, the most recent being the Change Healthcare Attack that effectively shut down the nation’s largest healthcare payment system. These attacks are not one-off events; they are just the latest instance of ne’er-do-well youths lurking in the darkness of an after-hours theme park carport and cutting a hole in the fence.
Locally deployed LLMs allow us to create an air-gapped island for our dinosaurs, safe from the bad decisions of a misspent youth / state-sponsored cyber-attack (same, same). Deploying self-contained LLMs or LLMs integrated within a preexisting containerized environment allows the federal enterprise to leverage a technology that is already widely available to the public and is revolutionizing how we interact with and generate language. These sophisticated algorithms, trained on massive datasets of text and code, possess remarkable capabilities in areas like text generation, translation, and question-answering. However, their application extends beyond general-purpose tasks, offering promising avenues for specialized sectors like government policy development.
Our very own dinosaur islands. Unlike traditional LLMs reliant on vast internet-sourced data, federated LLMs operate entirely offline, ensuring the highest level of privacy and security. This is particularly crucial when dealing with protected information, such as Personally Identifiable Information (PII).
Imagine a scenario where a health agency leverages an LLM for policy development. This LLM, trained on a curated and continually updated dataset of relevant materials such as NIST guidance, Executive Orders, and past policies, could assist in:
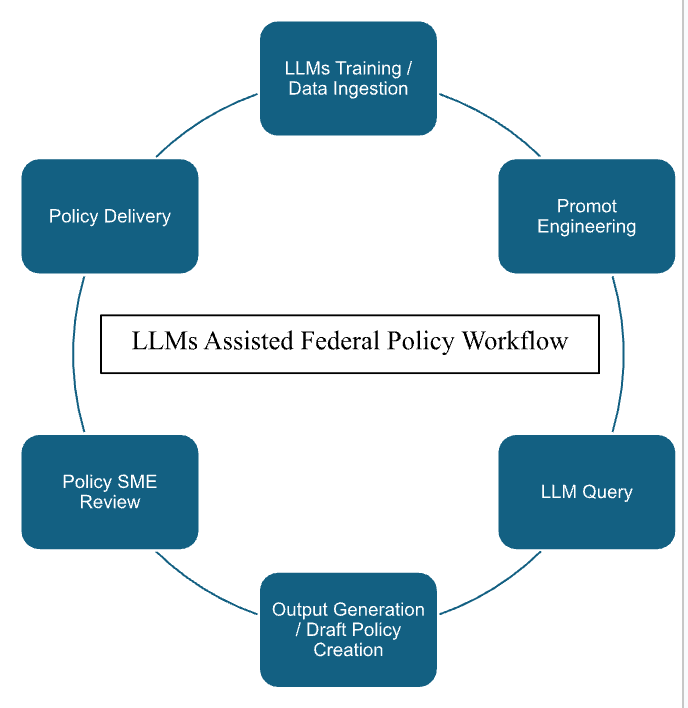
- Drafting new or updated policies: By prompting the LLM with specific parameters, analysts can generate initial policy drafts based on the model's understanding of existing regulations and precedents.
- Enhancing efficiency: The LLM can significantly reduce the time and resources traditionally required for research, writing, formatting, and revising policy documents. LLMs will likely have a similar impact on policy production to the one they currently have on the early adopters in code creation. That is, “inner loop” resource expenditure (time spent creating the initial code) has greatly decreased with AI assistance. However, “outer loop” tuning has increased. This signals a shift of expertise from creation to tuning. As such, as LLMs are implemented in federal policy workflows SME’s will likely spend most of their time reviewing content for accuracy and quality, as opposed to content creation (as they do today). Early reviews of coding workflows indicate that there is a 10% to 20% increase in output using AI assistance. Using this data as a benchmark and adjusting for the less technical nature of policy creation, a policy workflow may conservatively increase production by more than 30%.
- Maintaining accuracy and consistency: The LLM ensures adherence to established guidelines and formatting, minimizing the risk of errors or inconsistencies across policies.
- Reduced Hallucination Rate: An LLM that is trained on a specific set of quality data will reduce the hallucination rate. In addition, input query templates and exercising outcome supervision can be built into the models to provide human experts with the best output.
The application of dinosaur islands… or rather segregated LLMs in federated spaces, holds immense potential for government agencies and other organizations dealing with sensitive data. Government experts have felt for too long that they were the dinosaurs on the island, far removed from the mainland of modern technology available to everyone else. Our government experts should be augmented by the best technology we can offer, so rather than put an ocean between them and AI tools, let’s put the LLMs on an island. Freeing LLMs from the cloud and allowing them to run locally on specific datasets will transform how policies are written and how human experts spend their time. LLMs in federated spaces can be built and deployed securely, even in regulated environments. The future of the LLM is not necessarily large.
Nick Reese is a research associate for Emerging Technology at GoTech and former Director for Emerging Technology Policy at the Department of Homeland Security (2019-2023). Read Nick’s Full Bio
Chris Bodley is an emerging technology integrator and national security expert with over 15 years of experience in Counter-Malign Influence, Counterterrorism, and Counterintelligence Operations. He is currently a Cybersecurity Program Director, supporting the US government with enterprise solutions and modernization efforts.