Nov 5, 2024
From Cloud to Edge: Why Financial Services Are Moving Toward On-Premise AI
The financial services industry has long been at the forefront of adopting new technologies to enhance efficiency, improve customer experience, and manage risk.
November 5, 2024
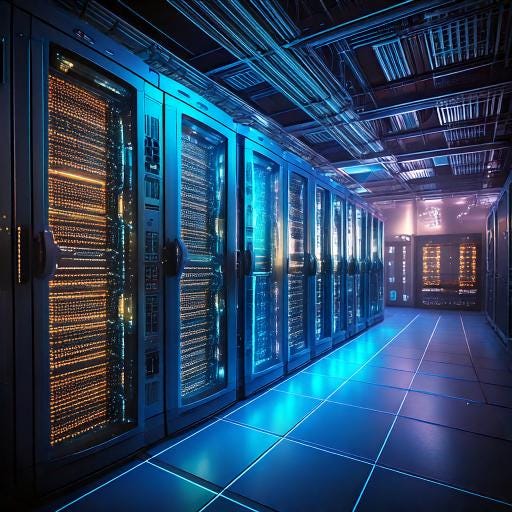
The financial services industry has long been at the forefront of adopting new technologies to enhance efficiency, improve customer experience, and manage risk. Cloud computing, with its promise of scalability, flexibility, and cost-effectiveness, was initially embraced by many financial institutions as a means to modernize their IT infrastructure. However, as the sector continues to evolve, there is a growing recognition of the limitations and risks associated with cloud-based solutions, particularly in the context of data privacy, security, and regulatory compliance. As a result, there is a noticeable shift from cloud to on-premise or edge AI solutions, where the computational workload is processed closer to the data source. This article explores the factors driving this shift, the benefits of on-premise AI for financial services, and the path forward for institutions looking to embrace this paradigm.
A Roadmap to AI Adoption
Develop a Strategic AI Roadmap
Invest in Infrastructure and Talent
Ensure Robust Data Governance and Security
Foster Collaboration and Knowledge Sharing
Continuously Monitor and Optimize AI Deployments
The Shift Toward On-Premise and Edge AI Solutions
The limitations and risks associated with cloud-based AI solutions have driven a growing shift toward on-premise and edge AI solutions. On-premise AI refers to AI systems that are deployed within an organization’s own infrastructure, while edge AI involves processing data closer to its source, such as on local servers or devices, rather than in a centralized cloud environment. Several factors are driving this shift:
Data Privacy and Sovereignty Concerns : One of the primary drivers of the shift toward on-premise AI is the need to maintain control over sensitive data. Financial institutions handle vast amounts of highly sensitive information, including personally identifiable information (PII), financial records, and transaction data. Regulatory frameworks such as the General Data Protection Regulation (GDPR), the California Consumer Privacy Act (CCPA), and sector-specific guidelines like the Payment Card Industry Data Security Standard (PCI DSS) impose strict requirements on how data is handled, stored, and processed. On-premise AI solutions allow organizations to retain full control over their data, reducing the risk of data breaches and ensuring compliance with data sovereignty laws.
Enhanced Security and Risk Management : The financial sector is a prime target for cyberattacks, given the high value of the data it handles. Cloud environments, while generally secure, are not immune to breaches and cyber threats. On-premise AI solutions enable financial institutions to implement more stringent security measures, such as encryption, access controls, and network segmentation, tailored to their specific risk profiles. This reduces the attack surface and allows for more proactive risk management, ensuring that sensitive data is protected from potential threats.
Regulatory Compliance and Auditability : Compliance with regulatory requirements is a top priority for financial institutions. On-premise AI solutions provide greater transparency and control over data flows, making it easier to demonstrate compliance with regulatory standards. Additionally, they offer more robust audit trails and logging capabilities, enabling institutions to monitor and report on data access and usage in a manner that satisfies regulatory scrutiny.
Reduced Dependency on Third-Party Providers : Cloud-based AI solutions often involve reliance on third-party providers, which can introduce additional risks, including vendor lock-in, service outages, and data migration challenges. On-premise AI solutions reduce this dependency, providing organizations with greater control over their technology stack and ensuring business continuity in the event of a service disruption.
Performance and Latency Advantages : On-premise and edge AI solutions can offer performance and latency advantages over cloud-based solutions, particularly for real-time applications. In areas such as fraud detection, algorithmic trading, and risk management, the ability to process data and generate insights in real-time is critical. By deploying AI closer to the data source, institutions can reduce latency, enhance performance, and make faster, more informed decisions.
Share
The Benefits of On-Premise AI for Financial Services
The shift from cloud to on-premise AI is not merely a response to regulatory and security concerns; it also offers several strategic benefits that can enhance the operational efficiency and competitiveness of financial institutions.
Greater Control Over Data and AI Models : On-premise AI solutions provide organizations with greater control over their data and AI models, allowing for more tailored and flexible deployments. This is particularly important for financial institutions that need to customize AI models to fit their unique risk management, compliance, and operational requirements. By maintaining control over AI models, institutions can ensure that they align with internal policies, regulatory standards, and ethical guidelines.
Cost Efficiency in the Long Term : While cloud solutions offer an attractive pay-as-you-go model, costs can quickly escalate as data volumes and processing requirements grow. On-premise AI solutions may require higher initial capital investment in infrastructure, but they can be more cost-effective in the long term. Institutions can avoid ongoing subscription fees, data transfer costs, and potential price increases by cloud providers, ultimately reducing the total cost of ownership.
Enhanced Data Governance : Data governance is a critical concern for financial institutions, particularly in the context of AI. On-premise AI solutions allow organizations to establish more robust data governance frameworks, ensuring that data is properly managed, secured, and utilized. This is especially important for institutions that need to ensure data lineage, integrity, and quality across complex and distributed environments.
Support for Hybrid and Edge Deployments : On-premise AI solutions can support hybrid deployments, where some workloads are processed on-premises while others are managed in the cloud. This flexibility allows financial institutions to leverage the benefits of both environments, optimizing their AI infrastructure for specific use cases. For example, sensitive data can be processed on-premises to ensure privacy and security, while less sensitive workloads can be handled in the cloud to take advantage of scalability and cost savings.
Facilitation of Real-Time Decision-Making : In areas such as trading, fraud detection, and customer experience management, real-time decision-making is crucial. On-premise and edge AI solutions enable financial institutions to process data and generate insights closer to the source, reducing latency and enhancing the speed and accuracy of decision-making. This capability can provide a competitive advantage in fast-paced financial markets where milliseconds can make a difference.
Challenges and Considerations for On-Premise AI Adoption
While the benefits of on-premise AI for financial services are clear, there are also challenges and considerations that institutions must address to ensure successful adoption.
Infrastructure and Maintenance Costs : On-premise AI solutions require significant investment in hardware, software, and IT infrastructure, as well as ongoing maintenance and support. Financial institutions must carefully evaluate the total cost of ownership and ensure that they have the necessary resources and expertise to manage on-premise AI deployments effectively.
Talent and Expertise : Deploying and managing on-premise AI solutions requires specialized talent, including data scientists, AI engineers, and cybersecurity experts. Financial institutions must invest in building multidisciplinary teams that can effectively manage these systems, ensuring that they are aligned with the organization’s risk management, compliance, and business objectives.
Scalability Challenges : While on-premise AI solutions offer greater control and security, they may face scalability challenges as data volumes and processing requirements grow. Institutions must carefully plan their infrastructure to ensure that it can scale to meet future needs without compromising performance or security.
Integration with Existing Systems : Financial institutions often operate in complex IT environments with legacy systems, third-party applications, and disparate data sources. Integrating on-premise AI solutions into these environments can be challenging and may require significant customization and development work. Institutions must ensure that their AI solutions are compatible with existing systems and can be seamlessly integrated into their workflows.
Subscribe now
A Path Forward: Embracing On-Premise AI in Financial Services
The shift from cloud to on-premise AI represents a significant evolution in how financial institutions leverage AI to drive innovation, manage risk, and enhance customer experience. To successfully embrace on-premise AI, financial institutions should consider the following steps:
Develop a Strategic AI Roadmap : Institutions should develop a strategic roadmap for AI adoption that aligns with their business objectives, risk management frameworks, and regulatory requirements. This roadmap should identify key use cases for on-premise AI, assess the infrastructure and talent needs, and outline a phased implementation plan.
Invest in Infrastructure and Talen t: Building a robust on-premise AI capability requires investment in both infrastructure and talent. Financial institutions should prioritize investments in high-performance computing, data storage, and cybersecurity infrastructure, as well as in hiring and training the necessary talent to manage AI deployments effectively.
Ensure Robust Data Governance and Security : Data governance and security must be at the forefront of any on-premise AI strategy. Institutions should establish comprehensive data governance frameworks that address data privacy, security, quality, and compliance. Additionally, they should implement robust security measures, such as encryption, access controls, and network segmentation, to protect sensitive data from potential threats.
Foster Collaboration and Knowledge Sharing : The financial services industry is highly dynamic, and institutions can benefit from collaborating with peers, technology providers, and regulators to share insights, best practices, and standards for on-premise AI adoption. Collaboration can help institutions stay ahead of emerging risks and ensure that their AI strategies are aligned with industry trends and regulatory developments.
Continuously Monitor and Optimize AI Deployments : On-premise AI solutions require continuous monitoring and optimization to ensure that they deliver the desired outcomes. Financial institutions should establish performance metrics and monitoring frameworks to track the effectiveness of their AI deployments and make data-driven adjustments as needed.
The shift from cloud to on-premise AI represents a strategic evolution for financial services institutions looking to leverage the power of AI while ensuring data privacy, security, and regulatory compliance. By embracing on-premise AI solutions, financial institutions can enhance their data governance, reduce dependency on third-party providers, and gain greater control over their AI models and data. However, successful adoption requires careful planning, investment, and collaboration. By taking a proactive and strategic approach, financial institutions can position themselves to thrive in an increasingly digital and data-driven world.
To stay up to date with our work, please follow us on LinkedIn and visit our website . To learn more about the services we offer, please visit our product page.
Leave a comment