Apr 3, 2025
Solving the AI Mystery
This is how successful AI implementation is done.
April 3, 2025
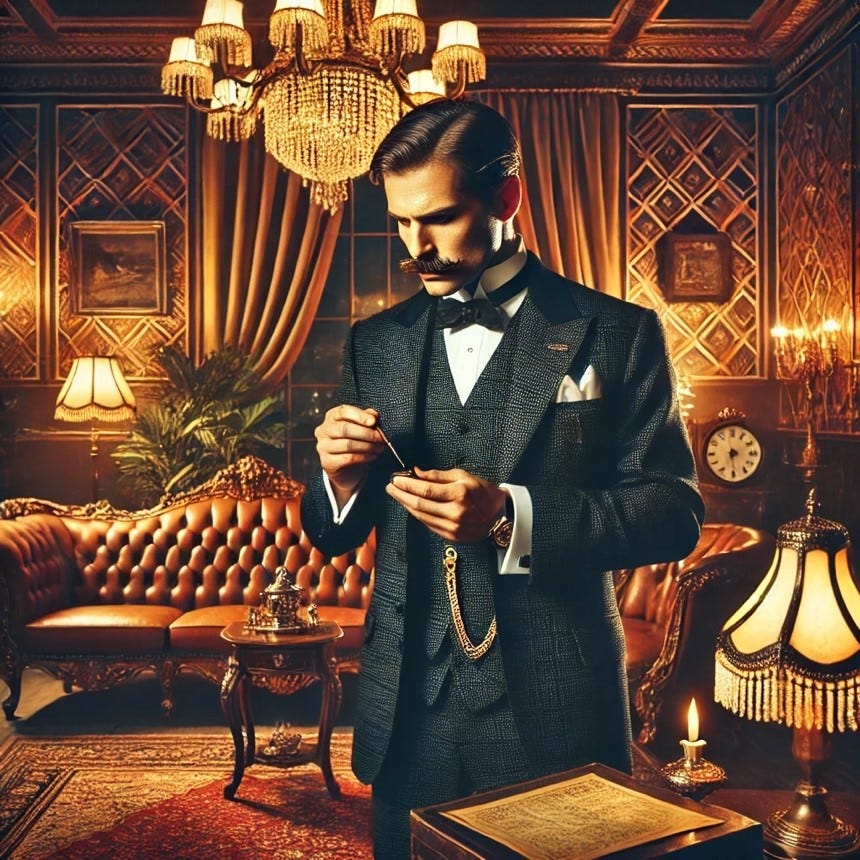
The canon of literature featuring Hercule Poirot spans 33 novels and 51 short stories starting in 1920 and ending in 1975. The slight and often seasick Belgian detective was made famous by Agatha Christie as he solved mysteries the world over whether on long haul trains or in European cities. In conference rooms and board rooms across the country, there are probably business leaders wishing they had their own in-house Hercule Poirot. How to implement AI into the right part of your business can feel like a mystery, perhaps even one beyond Detective Poirot. Identification of use cases, privacy considerations, data wrangling, workforce training, and so many other factors can play into the success or failure of an AI implementation. But we should not mistake Hercule for a clairvoyant. He couldn’t read minds or see the future. He followed the clues and saw signals in the small details that were overlooked by others.
This is how successful AI implementation is done. All we need is to look at the right details and build a plan to increase productivity , customer value, and economic growth.
A Personal Affair and Style
What makes AI implementation so daunting is that there is no playbook that an organization can follow that will lead it to success. More broadly, the playbooks with which we are comfortable do not apply equally to AI such as playbooks for IT or cybersecurity. Ultimately, AI comes down to a highly personal choice. Asking SHOULD the organization use AI and if so, under what conditions? This is an exercise in use case identification and the use cases will vary across sectors, industries, and individual organizations. Considering use cases requires organizations to think critically about their processes, value propositions, and ethics among other things. The below list would give Detective Poirot the first clues he would need to unravel this mystery. Imagine him picking up a scrap of paper with these words written on it from the smoldering embers of a fire ignited to throw him off the trail.
Automation: Are there processes that can be automated to increase speed, efficiency, or return time to your skilled workforce?
Workforce Augmentation: Would your skilled workforce be more productive or more accurate if they had data-driven insights?
Customer Delivery: Can you improve customer delivery through finding efficiencies or automating certain processes?
Marketing: Can you enter a new market or reach more target customers?
Ethics: Is customer privacy important to your organization?
Peril at Data House
The next issue that can cause delays in effective and successful AI implementation is how to structure the data around the identified use case. Much like investigating a complex murder, a single clue will not lead you to the answer you seek. The identification of the use case(s) will help you begin to understand the problem but will not solve it. The next consideration is how to structure the data you have in house or to which you have access to address the use case. Assuming the organization has an AI governance structure and the correct policies in place, bringing the right data to bear will be the difference in unique customer value creation and a mediocre improvement in processes. This structure will help you figure out how you can do more than just automate but find efficiencies that will drive real value in your organization. The next clue in our case is how to structure a data strategy around your use case.
Foundation: Foundational data should be structured or unstructured data that is directly relevant to your use case.
Example : A dataset of all trades for a financial services firm.
Foundation Adjacent: Largely unstructured data that provides the context for the foundational data relevant to the use case.
Example : Policy documents, regulations, court filings, news articles, social media feeds, and emails that provide context for the structured foundational data.
Best in Class Data: Structured or unstructured data that provides an example of best in class for the use case you identified. This could also be data that provides examples or WORST in class.
Example : Historical data on legal cases won or lost in the same legal area you are working on for a law firm.
The largest dataset will be the best-in-class data. Ideally, these data will cover multiple decades to give the AI the ability to recognize when something is going well in your use case and when something is not. The AI used in your use case is frankly only as good as the way you train it, and this structure allows you to train it directly on the output of your use case, give it context, then give it something to measure against. This structure creates a historical benchmark of past performance against which future performance can be measured. If the performance is falling below the benchmark, adjustments need to be made. What adjustments? That depends on the use case, but this structure will give the AI what it needs to find the answers like giving Hercule Poirot the address of the murder suspect.
Data Man’s Folly
Exactly how to implement AI into an organization is too big a question. The question must be broken down into smaller pieces the way a Belgian detective might break down a murder scene. Instead of asking,
“How can we implement AI?”
We should ask, “What use cases exist inside my organization where I want to drive efficiency or value?”
The next question should ask what data are available. The answer to that might also be too large so again this should be broken down into the pyramid structure above. Finally, the question about vendors should be asked. This question comes down to your organizations prioritization around three questions:
Time to value
Privacy
Customization
Share
Time to value asks you how long you are willing to wait for the solution. It also asks you how broad or targeted a solution you want to purchase. Many of the largest providers are looking for ways to resell the solution they’ve already sold a thousand times at the same scale, so the margins remain high. The implementation will come with months of work before a usable prototype is available. This may not work for every organization. Seeking competitive advantage may not afford an organization this much time. Considering the time to value of any vendor is a key issue.
One of the most significant differentiators is how your data, or the data of your customers, is handled. If you operate inside a regulatory envelope, or if you simply have data privacy as a part of your organizational ethics or value proposition, you will need to consider how the data is handled. Can you keep the data inside your secure environment? What about on premise? How can you protect the data that is being used by your AI? Ask this question of your vendor then ask it again.
A real folly in AI implementation is buying something that was built for another use case and maybe, sort of fits your use case, but not really.
Having real value from AI means having AI that was built for your purpose. The largest of the large language models that are trained on the entirety of digitized human knowledge making them the epitome of generalists. You may not need a generalist. You may need a system that was built by experts in your domain and understands your unique approach to your business. Don’t fall into the generalization trap.
It doesn’t take Hercule Poirot to figure out how to use this framework to drive real AI implementation in your organization. Identify your use cases, structure your data strategy, and question your vendors. Make the problem smaller and use those small bites to narrow down the ideal fit. AI is not monolithic and nor is your industry. Your future success in an AI-driven world depends on your ability to implement an AI solution that was built for your, preserves your privacy, and increases the productivity of your workforce. Perhaps Detective Poirot will not have to get on that trans-Atlantic ship after all.
Connect with us: Substack , LinkedIn , Bluesky , X , Website
To learn more about the services we offer, please visit our product page.
Nick Reese is the cofounder and COO of Frontier Foundry and an adjunct professor of emerging technology at NYU. He is a veteran and a former US government policymaker on cyber and technology issues. Visit his LinkedIn here .
This post was edited by Thomas Morin, Marketing Analyst at Frontier Foundry. View his LinkedIn here .
Subscribe now
Leave a comment